AI Workflow Automation vs Traditional Automation: What Business Leaders Need to Know
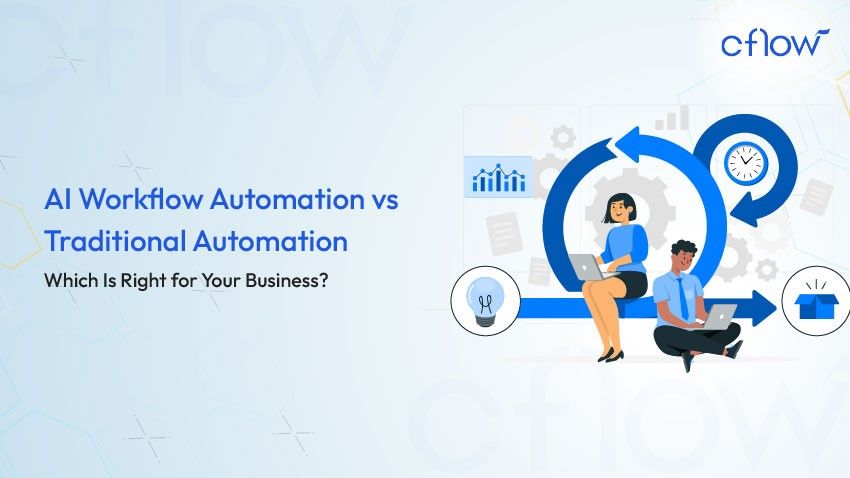
Key Takeaways
- AI workflow automation adapts and learns from data, unlike rule-based traditional systems that require manual updates for each change.
- Traditional automation improves repetitive task execution but lacks decision-making, pattern recognition, or real-time adaptability.
- AI-driven automation enhances operational agility, customer experience, and predictive analytics in dynamic environments.
- AI-powered workflow automation tools like Cflow combine rule-based reliability with adaptive intelligence to streamline complex workflows.
Automation has been instrumental in driving efficiency across industries for decades. As the global workflow automation market is projected to reach USD 23.77 billion by 2025 and USD 37.45 billion by 2030 (CAGR of 9.52%), the conversation around AI Workflow Automation vs Traditional Automation has become increasingly relevant for businesses seeking scalable and intelligent solutions.
Businesses first embraced traditional automation to streamline repetitive processes, eliminate manual errors, and reduce operational costs. While effective, these systems are limited by their rigidity—they can only operate within the parameters defined by developers.
Today, organisations are moving beyond static workflows by embracing AI workflow automation. Powered by machine learning and predictive analytics, AI introduces intelligence into workflows. These systems can learn from data, adapt to new inputs, and make decisions with minimal human intervention.
Before diving into platforms or tools, it’s essential for business leaders to understand the difference between AI and traditional automation. AI doesn’t replace traditional systems—it enhances them, offering a hybrid solution where structured and unstructured tasks are both optimised.
What is Traditional Automation?
Traditional automation refers to rule-based systems that operate based on predefined logic and conditions. These workflows follow set instructions to perform tasks consistently. Whether it’s macros, scripts, workflow engines, or RPA, these tools are designed to reduce human effort for repetitive, high-volume tasks.
A simple example is an invoice approval system that routes documents based on the amount: anything under $5000 is auto-approved; anything over is flagged for review. This system doesn’t analyse context—it just follows rules.
The benefits of this approach include speed, reliability, and reduced costs. It’s also relatively easy to implement and scale for structured environments. However, when inputs vary or exceptions occur frequently, its limitations become evident.
This contrast between AI vs rule-based automation highlights how AI’s contextual capabilities outperform rigid systems when adaptability is needed.
What is AI Workflow Automation?
According to McKinsey, 21% of organisations using generative AI have fundamentally redesigned at least some workflows, indicating a significant shift towards AI integration.
AI workflow automation introduces intelligence into process management. Unlike rule-based systems, AI workflows leverage machine learning, natural language processing, and predictive analytics to make real-time decisions and evolve with each interaction.
These systems can analyse customer sentiment, classify documents, identify anomalies, and even detect fraud. For instance, a support ticketing system using AI can scan incoming emails, identify urgency based on tone and keywords, and route them to the appropriate team instantly.
The power of machine learning in workflow automation lies in continuous improvement. As more data flows through the system, it becomes better at recognising patterns and adjusting responses.
AI workflows are ideal for managing unstructured data, evolving scenarios, and tasks requiring judgment—something traditional automation simply cannot match.
Key Differences Between AI Workflow Automation and Traditional Automation
Aspect | Traditional Automation | AI Workflow Automation |
Approach | Rule-based systems that follow fixed, pre-defined logic | Data-driven systems that adapt based on machine learning models and real-time data |
Flexibility | Limited to the scenarios defined at setup, rigid and difficult to adapt | Highly flexible; adjusts to changes in data, user behaviour, and patterns |
Learning Ability | Cannot learn from experience; operates only within its coded parameters | Continuously learns from data through ML algorithms, improving accuracy and decisions over time |
Setup Requirements | Quick to deploy with minimal data; suitable for straightforward processes | Requires historical data and training phases; initial setup takes more time |
Use Cases | Works best for repetitive, rule-bound tasks like invoice approvals or sending notifications | Ideal for tasks involving unstructured data and variable inputs like fraud detection or intelligent routing |
Example | Sends automated email reminders at fixed intervals | Analyses content and urgency of emails to prioritise or auto-respond intelligently |
When to Use Traditional Automation vs AI Automation
The intelligent automation vs conventional automation debate isn’t about choosing one over the other—it’s about applying the right tool for the right task. Traditional automation is best suited for high-volume, low-variability tasks that involve structured data inputs and predictable decision-making. A common example is invoice validation, where the rules are fixed, and outcomes are repeatable.
In contrast, AI workflow automation excels in scenarios involving unstructured or semi-structured data. It is ideal for workflows that require context, adaptability, or even sentiment analysis. Use cases include customer service interactions, regulatory compliance tasks, fraud detection, and HR screening—areas where human-like judgment or pattern recognition plays a critical role.
The most successful organisations today don’t pick sides. Instead, they combine both approaches—leveraging the speed and reliability of traditional automation alongside the intelligence and flexibility of AI. This blended strategy ensures a more agile, scalable, and future-ready operations model.
Challenges of Traditional Automation
Traditional workflow automation has played a key role in streamlining routine tasks. However, as business needs evolve, its limitations become more apparent. Below are the main challenges:
1. Static and Rule-Based Logic
Traditional systems rely on rigid, predefined rules. They struggle to handle dynamic scenarios, exceptions, or real-time decision-making, making them ill-suited for complex or adaptive workflows.
2. Limited Handling of Unstructured Data
These tools perform best with structured inputs like forms or spreadsheets. Dealing with emails, scanned files, or free-text data requires manual workarounds or advanced scripting.
3. High Dependence on IT Teams
Workflow changes, integrations, or troubleshooting often require developer support. This slows down innovation, increases IT burden, and limits business user autonomy.
4. No Learning or Adaptability
Traditional automation doesn’t learn from past outcomes. It cannot self-correct or optimise processes over time, leading to stagnant efficiency and recurring manual oversight.
5. Poor Resilience to Change
Even minor updates to data formats or system rules can disrupt workflows. These fragile setups require continuous maintenance, reducing scalability and increasing operational risk.
Use Cases Across Industries
AI-driven process automation is revolutionising operations across sectors. By enhancing traditional workflows with intelligence, businesses are improving accuracy, adaptability, and speed at scale.
1. Manufacturing
Traditional automation in manufacturing relies on robotic assembly lines to handle repetitive, pre-defined tasks. With AI, predictive maintenance uses real-time sensor data to anticipate equipment failures and minimise downtime.
2. Finance
Rule-based systems in finance handle compliance and transaction validation effectively. AI takes it further by enabling real-time fraud detection and credit risk analysis based on behavioural patterns.
3. Healthcare
Automation in healthcare helps with scheduling and sending reminders. AI enhances this by analysing patient history and imaging data to support diagnostic decisions and improve outcomes.
4. Human Resources
Traditional HR tools parse resumes using keyword filters. AI empowers recruitment by scoring candidates based on behavioural insights and predicting future performance through advanced analytics.
Benefits of AI Workflow Automation
AI is reshaping how businesses operate by bringing intelligence, speed, and adaptability into everyday processes. Below are five key benefits of adopting AI in workflow automation:
1. Smarter Decision-Making
AI uses context, intent, and real-time variables to make human-like decisions. It improves workflows like customer support by routing requests intelligently. This reduces escalations, shortens response times, and boosts resolution rates.
2. Improved Accuracy
By learning from historical data, AI detects anomalies and reduces errors. It also standardises data across systems for better compliance. This ensures higher accuracy in finance, healthcare, and legal operations.
3. Dynamic Process Adaptation
AI updates workflows automatically based on changing inputs and user behaviour. Unlike rigid traditional systems, it enables real-time agility and scale. Businesses can adapt faster to policy shifts or market demands.
4. Data-Driven Optimisation
AI constantly evaluates workflows to spot inefficiencies and redundant steps. It suggests optimisations that improve throughput and cycle times. This ensures operations remain aligned with evolving business goals.
5. Enhanced Customer Experience
AI delivers faster, more tailored service by analysing user history and preferences. From chatbots to recommendations, it personalises each interaction. This builds trust, enhances satisfaction, and boosts brand loyalty.
End-to-end workflow automation
Build fully-customizable, no code process workflows in a jiffy.
Technologies and Techniques Used in AI Workflow Automation
AI workflow automation integrates several advanced technologies to enable intelligent, adaptive, and scalable operations. Below are the key components driving this transformation:
1. Machine Learning (ML)
ML algorithms allow systems to learn from data patterns and improve over time. From fraud detection to invoice categorisation, ML supports workflows that evolve with each interaction.
2. Natural Language Processing (NLP)
NLP helps automation tools understand and process human language. It powers chatbots, sentiment analysis, and document classification by interpreting unstructured text in real time.
3. Computer Vision
This technology enables systems to interpret visual content. It’s used in document scanning, image-based approvals, and OCR (Optical Character Recognition) for extracting data from physical forms.
4. Predictive Analytics
By analysing historical trends and current performance, AI forecasts outcomes such as customer churn or payment delays. This allows workflows to trigger preventive actions before issues arise.
5. Robotic Process Automation (RPA) + AI
Combining rule-based RPA with AI creates intelligent automation. RPA handles repetitive tasks, while AI manages judgment-driven processes, enabling end-to-end workflow intelligence.
6. Integration APIs and Webhooks
APIs and webhooks connect AI systems with CRMs, ERPs, and cloud tools. They enable real-time data exchange and ensure that workflows remain synchronised across platforms.
How AI Enhances Traditional Automation
AI doesn’t replace traditional systems—it enhances them by injecting intelligence and adaptability into otherwise static workflows. Below are four ways this synergy creates smarter business operations.
1. AI + RPA: From Static Bots to Intelligent Assistants
Robotic Process Automation (RPA) performs repetitive, rule-based tasks efficiently. When AI is added, these bots evolve into intelligent agents. They can now interpret natural language, analyse the context, and make dynamic decisions, handling tasks like email classification, compliance reviews, or customer query resolution with human-like insight.
2. AI + OCR: Intelligent Data Extraction
Traditional OCR reads text from scanned documents. With AI, OCR systems learn to recognise layouts, fix formatting issues, and extract only relevant data. This is especially valuable in industries like finance or healthcare, where automating document processing (e.g., invoices and claims) drastically improves speed and accuracy.
3. AI + Alerts: Predictive Monitoring for Proactive Action
Basic automation alerts flag issues based on static thresholds. AI enhances this by predicting equipment failure or data anomalies before they occur. For example, in manufacturing, AI models analyse sensor data in real-time and trigger preventive workflows to reduce unplanned downtime.
4. AI + Traditional Systems: Seamless Operational Intelligence
Integrating AI with existing platforms results in workflows that evolve. AI adapts to new patterns, continuously improves outcomes, and empowers teams to make data-driven decisions. This blend delivers scalable, resilient automation that goes beyond speed, unlocking real-time responsiveness and intelligent process execution.
Future AI Workflow Automation Trends to Watch
AI is evolving fast, but the future promises an even more radical shift. Beyond today’s automation, we’re entering an era where workflows will be self-generating, emotionally adaptive, and deeply personalised. Here are five next-gen trends poised to redefine the landscape.
1. Prompt-Driven Autonomous Workflow Creation
In the near future, AI will generate workflows from a simple prompt. Users will describe goals in plain language (e.g., “automate employee onboarding”), and AI will instantly build the logic, conditions, and integrations—no setup needed.
2. Emotionally Aware AI Agents
Future AI will analyse tone, facial cues, and voice modulation to detect emotions and modify workflows accordingly. An upset customer may trigger a faster escalation route, creating more humane, responsive automation.
3. AI Simulation Before Workflow Deployment
Before launching, AI will simulate workflows across data scenarios to predict errors, bottlenecks, or compliance issues. These digital “trial runs” will ensure accuracy and eliminate risks, especially for complex, high-impact processes.
4. Personalised Workflows for Each User
Workflows will adapt not just to business needs but to individual users. Based on behaviour and role, AI will customise tasks, notifications, and dashboard layouts, maximising efficiency for every employee.
5. Decentralized Autonomous Workflows (DAWs)
Powered by blockchain and smart contracts, DAWs will execute securely across ecosystems without central control. Ideal for vendor management, procurement, and public sector processes that require trust and traceability.
Ethical Considerations and Bias Mitigation in AI Automation
- AI systems can unintentionally reinforce bias if trained on flawed or non-representative data, especially in high-stakes areas like hiring, healthcare, and finance.
- Lack of transparency in decision-making leads to compliance risks and loss of stakeholder trust, particularly in regulated industries.
- Bias in AI can result in unfair outcomes, such as discriminatory hiring practices or unequal healthcare diagnostics.
Key mitigation strategies include:
- Using diverse and representative training datasets.
- Conducting regular audits of algorithms for fairness.
- Keeping humans in the loop for critical decision points.
- Ensuring all AI decisions are traceable and explainable.
Responsible AI isn’t just compliance—it’s a trust builder, helping companies position themselves as ethical and future-ready.
Strategies to Successfully Implement AI Workflow Automation
A recent survey indicates that 74% of current users plan to increase AI investments in the next three years, reflecting growing confidence in AI’s value.
Implementing AI workflow automation requires a structured, cross-functional approach that balances technology, people, and process.
Begin by auditing current workflows—map out routine, rule-based processes and flag those with decision-making gaps or inefficiencies. These are ideal candidates for AI.
Key strategies for effective implementation include:
- Set clear objectives: Define what success looks like—reduced turnaround time, improved accuracy, or lower costs. This will guide tool selection and performance tracking.
- Start with pilot projects: Choose a non-critical workflow to test AI functionality. Use results to validate your approach and fine-tune the setup.
- Ensure data quality: AI thrives on clean, labelled, and well-structured data. Consolidate fragmented data sources before training models.
- Choose integration-friendly tools: Select AI solutions that work well with existing systems via APIs or connectors to avoid silos.
- Invest in training and change management: Upskill teams, build internal champions, and communicate clearly to manage resistance.
With the right foundation, AI can deliver long-term, scalable transformation.
AI Workflow Automation with Cflow
Cflow, a no-code workflow automation platform, now integrates Seyarc AI to revolutionise how workflows are built and deployed. Instead of manually configuring steps or designing flowcharts, users can describe their process in natural language or simply upload a visual diagram, and Cflow takes care of the rest.
With prompt-based workflow generation, users provide a basic text prompt, and Seyarc AI interprets the intent to automatically generate a ready-to-use workflow. This removes the need for technical expertise or time-consuming design work. Alternatively, users can upload a visual process diagram, which the AI scans and replicates inside Cflow, turning static visuals into interactive, executable workflows.
This dual capability dramatically reduces setup time, allowing businesses to move from concept to execution in minutes. Whether it’s designing an approval process or digitising an onboarding journey, Cflow with Seyarc AI empowers organisations to achieve operational automation with minimal input and zero coding.
Focused on speed, simplicity, and smart automation, Cflow’s AI-driven features help teams streamline workflow creation, making it easier to adopt digital transformation at scale.
Conclusion
The AI-powered automation market is projected to reach $25 billion by 2027. AI workflow automation is not a replacement for traditional automation—it’s the next logical step forward. While rule-based systems laid the groundwork for operational efficiency, they lack the intelligence to adapt and scale in today’s fast-moving business environment. AI brings that missing layer of insight, flexibility, and continuous improvement.
By combining the reliability of traditional automation with the learning capabilities of AI, organisations can future-proof their operations, reduce bottlenecks, and deliver smarter outcomes.
Ready to evolve beyond static automation? Sign up for Cflow today and bring intelligence into every workflow.
FAQs
1. What is the main difference between AI workflow automation and traditional automation?
The core difference lies in adaptability. Traditional automation uses fixed, rule-based logic and works well for repetitive tasks with structured data. In contrast, AI workflow automation is data-driven and adaptive, using machine learning to handle unstructured data, make decisions in real-time, and improve continuously.
2. Can AI workflow automation fully replace traditional automation systems?
Not entirely. AI enhances rather than replaces traditional systems. While AI excels in dynamic, judgment-based tasks, traditional automation is still ideal for structured, repetitive operations. The most effective strategy combines both, allowing organisations to optimise performance across varied workflows.
3. What should companies consider before switching to AI workflow automation?
Businesses should evaluate data quality, workflow complexity, and integration readiness. AI systems need clean historical data, training time, and well-defined goals. Starting with pilot projects and choosing integration-friendly platforms like Cflow can ensure a smooth transition and better ROI.
What would you like to do next?
Automate your workflows with our Cflow experts.