How to Measure AI Workflow Automation Success
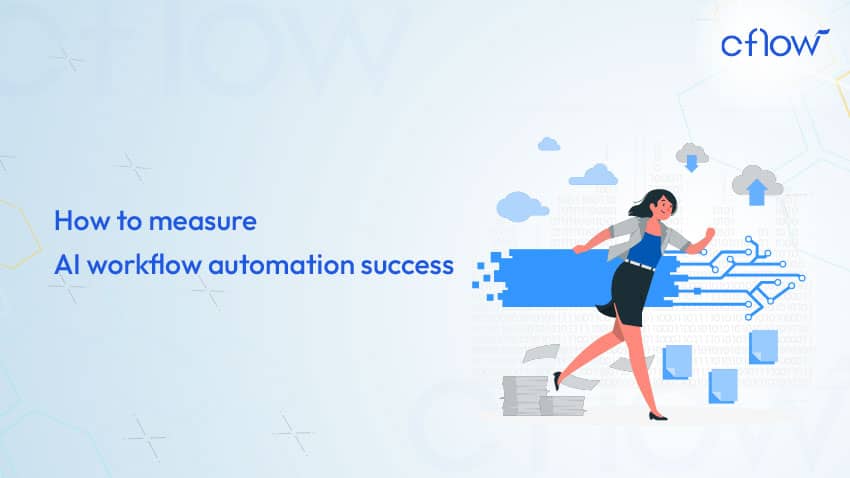
Key takeaways
- Measuring AI workflow automation success requires tracking both technical and business performance indicators aligned to company goals.
- Key metrics include cost savings, productivity gains, SLA compliance, and AI decision accuracy across workflows.
- Real-time dashboards, predictive analytics, and feedback loops help maintain accountability and optimise performance.
- Platforms like Cflow offer built-in analytics that simplify performance tracking, ROI visualisation, and continuous process improvement.
AI-powered workflow automation has moved from hype to a core component of digital transformation. Businesses that once saw AI as a future investment now recognise its necessity in streamlining processes, reducing overhead, and delivering real-time insights. However, success with AI doesn’t end with deployment. In fact, true success begins after implementation, when companies must evaluate performance, justify ROI, and optimise continuously.
Organisations that have advanced beyond the initial testing phase of intelligent automation report an average cost savings of 32%.
Measuring success provides clarity on what’s working, what needs improvement, and where AI is delivering measurable business value. With automation touching everything from procurement to HR to customer service, a well-defined measurement framework ensures your AI strategy drives real results.
This blog outlines how to assess effectiveness across operations, decision-making, and cost—while showcasing how platforms like Cflow make measurement seamless.
What Is AI Workflow Automation Success?
AI workflow automation success refers to the demonstrable achievement of business outcomes—like time savings, cost reduction, and improved accuracy—through AI-powered workflow tools. Success isn’t just a technical accomplishment; it’s a strategic one. The effectiveness of AI is judged by its contribution to operational efficiency, consistency, and competitive advantage.
AI workflow automation can increase productivity by up to 4.8 times and reduce errors by 49%.
An AI-enabled process should exhibit the following outcomes:
- Operational efficiency: Tasks completed faster, with fewer delays and dependencies.
- Cost-effectiveness: Reduction in staffing needs, manual effort, and rework.
- Accuracy and compliance: Reduced error rates and stronger adherence to policies.
- Smarter decision-making: AI offers contextual suggestions and escalations when needed.
- Scalability: Easily applies across teams or departments, without degradation in performance.
In practice, a finance department may track if invoice approvals are faster, require fewer manual interventions, and maintain or improve compliance after automation. The point is: success must be visible, measurable, and repeatable.
Why Measuring Success Is Crucial for AI Workflow Automation
Measuring success is not optional—it’s foundational. Without tracking progress and outcomes, you won’t know if automation is truly solving the problems it was meant to. Here’s why it’s critical:
- ROI Justification: Business leaders and stakeholders need clear evidence of value to justify continued investment or scaling of automation.
- Performance Assurance: AI models require regular validation to ensure they are working as expected. Drift in decision quality must be caught early.
- Optimisation Feedback Loop: Metrics help pinpoint where the workflow falters, enabling targeted improvements and better resource allocation.
- Strategic Clarity: Helps align AI automation projects with high-level business goals like revenue growth, customer satisfaction, or employee engagement.
A robust measurement framework ensures that AI efforts evolve with the business rather than operate in a silo. Measurement brings automation into alignment with performance culture.
Key Metrics to Measure AI Workflow Automation Success
Businesses using workflow automation save an average of $46,000 annually.
Effective measurement starts with knowing what to track. Metrics should be tailored to your business goals and use case. These fall into three essential categories:
1. Operational Metrics
These metrics evaluate the day-to-day efficiency of workflows:
- Turnaround Time (TAT): Measures how long it takes for a process or task to be completed from start to finish. A lower TAT after automation signals success.
- Cycle Time Reduction: The overall time reduction across process stages. This metric reveals delays eliminated by automation.
- Process Throughput: Indicates how many workflows or tasks are completed in a specific period. Higher throughput suggests more efficient operations.
- Error Rate: Tracks mistakes made in task execution. A significant drop in errors post-automation confirms enhanced reliability.
- Automation Coverage: Percentage of the entire process that is automated. The broader the coverage, the greater the potential benefits.
2. AI-Specific Metrics
These show how the AI element of automation performs:
- Decision Accuracy: Compares AI recommendations to ideal or human decisions. It confirms if AI is reliable.
- AI Escalation Rate: Measures how often AI refers tasks to humans due to uncertainty. A low but appropriate escalation rate indicates good calibration.
- Model Drift: Over time, AI models may lose effectiveness. Tracking performance over months helps catch this decline.
- Confidence Scores: Many AI systems assign scores to their decisions. Monitoring confidence score trends reveals decision stability.
- Feedback Loop Effectiveness: Evaluates whether AI systems are learning from user input or failing to adapt.
3. Business Impact Metrics
These link automation to broader business objectives:
- Return on Investment (ROI): Calculated by comparing automation benefits (time saved, errors avoided, costs reduced) to implementation and operational costs.
- Cost Savings: Includes reduced payroll from eliminated manual tasks, savings from error reduction, and compliance penalty avoidance.
- Time Savings: Measures time saved for employees, enabling them to focus on strategic tasks rather than routine ones.
- Customer Satisfaction (CSAT/NPS): Automation can lead to faster service and fewer errors, improving satisfaction scores.
- Employee Productivity: Looks at time reallocation and whether employees are doing more valuable work post-automation.
Tools and Methods to Track Automation Success
Your ability to measure success depends on the tools you use. Manual tracking with spreadsheets isn’t sustainable in the long run. To ensure consistency, accuracy, and agility in evaluating AI workflow automation, organisations must invest in scalable, integrated solutions that offer real-time insights and automated tracking. Let’s explore the three primary categories of tools that enable comprehensive performance measurement.
Workflow Automation Platforms with Built-in Analytics
Solutions like Cflow come with built-in analytics that are purpose-built for tracking workflow automation performance. These platforms eliminate the need for external configurations or integrations and empower users, regardless of technical proficiency, to track metrics in real time.
Key capabilities include:
- Custom Dashboards: Visualise KPIs across departments with filters based on roles, teams, or functions.
- SLA Alerts and Breach Tracking: Instantly flag delays or missed deadlines, allowing immediate corrective action.
- Process Timelines: Map out process stages visually to pinpoint exactly where bottlenecks are forming.
- Role-Based Reporting: Tailor reports based on decision-makers’ needs, from team leads to executives.
- Historical Trend Analysis: Review how process efficiency has evolved over time to support continuous improvement.
With these built-in tools, Cflow enables process owners and business leaders to visualise performance, identify lags, and course-correct without needing IT intervention.
Business Intelligence (BI) Integrations
For more complex environments or enterprises needing deeper insights, integrating workflow automation data into Business Intelligence (BI) platforms enables holistic analysis alongside other business data sources. This is particularly useful when automation metrics must be tied to revenue, supply chain KPIs, or workforce analytics.
Top BI tools include:
- Power BI: Enables cross-platform data integration and the creation of executive dashboards with drill-down capabilities.
- Tableau: Excels in creating dynamic visualisations that merge workflow metrics with CRM, ERP, and customer service data.
- Google Looker: Offers embedded analytics within web applications and collaborative features for cross-functional teams.
- Excel Dashboards: Still relevant for departments needing simple, agile reporting with minimal training or dependency.
These integrations expand visibility and allow leadership to tie automation outcomes directly to business goals, such as cost reduction, revenue growth, or compliance improvement.
User-Driven Feedback and Surveys
Numbers alone don’t tell the full story. People using the AI-powered workflows can provide valuable qualitative feedback that supports or challenges the quantitative findings. Human-centred feedback mechanisms allow businesses to track sentiment, ease of use, and AI decision acceptability.
Key feedback channels include:
- Employee Feedback Forms: Embedded into workflows to capture usability issues, time consumption, and clarity of automated actions.
- Customer Satisfaction (CSAT) Surveys: Measure the impact of automation on customer experience, such as ticket response time or delivery speed.
- AI Decision Reviews: Allow users to rate or correct AI decisions, thereby fine-tuning model performance over time.
Combined with analytics, these feedback mechanisms ensure a holistic view of AI workflow automation success.
Challenges in Measuring AI Workflow Automation Success
Despite its importance, measuring automation success comes with its own set of challenges that can distort or delay actionable insights. Organisations must recognise and proactively address these barriers to build a robust success measurement model.
1. Lack of Baseline Data
Organisations often rush to deploy AI workflows without first capturing baseline performance data. Without “before” benchmarks, it becomes difficult to quantify “after” results, leading to vague ROI discussions and missed improvement opportunities.
2. Inadequate Metrics
A narrow focus on financial savings may cause teams to overlook qualitative benefits like reduced stress, improved employee morale, and enhanced customer satisfaction. Effective measurement should include a mix of technical, business, and human-centric metrics.
3. AI Opacity (Black Box Problem)
Many AI models make decisions in ways that are difficult to explain, especially in complex processes. This lack of transparency—known as the black box problem—creates hesitation in trusting AI decisions and makes it harder to measure the model’s contribution accurately.
4. Disconnected Data Sources
Workflows often span across CRM, HRMS, ERP, and external platforms. When data isn’t centralised or easily integrated, generating accurate, end-to-end performance reports becomes a manual, error-prone task.
5. Stakeholder Misalignment
Different departments may define success differently. For instance, IT may focus on error reduction, while finance emphasises cost savings. Without cross-functional alignment, success metrics can conflict, leading to fragmented reports and misinformed strategy decisions.
Addressing these challenges early in the automation journey will ensure a more reliable and insightful measurement process.
End-to-end workflow automation
Build fully-customizable, no code process workflows in a jiffy.
Framework to Measure AI Workflow Automation Success
A structured framework brings consistency and repeatability to success measurement. It also ensures that evaluations are based on real business needs and align with strategic goals.
Step 1: Set Clear Objectives
Define the purpose of automation in clear terms. Is it to reduce turnaround times? Increase compliance accuracy? Improve employee experience? Objectives must be specific, measurable, and agreed upon by stakeholders.
Step 2: Select Relevant Metrics
Each objective should be tied to a set of relevant KPIs. For example, if the goal is to reduce turnaround time, then TAT, SLA compliance, and escalations should be tracked. Avoid data overload by focusing only on metrics that reflect impact.
Step 3: Establish Baselines
Capture existing performance metrics before implementing automation. Where baseline data is unavailable, historical averages or pilot project outcomes can serve as reference points.
Step 4: Automate Monitoring
Leverage automation tools like Cflow or BI dashboards to continuously collect and display data. Avoid manual tracking and ensure data is updated in real time or near-real time to maintain accuracy.
Step 5: Review, Optimise, Repeat
Success measurement is not a one-time task. Conduct quarterly or monthly reviews to assess results, recalibrate KPIs if needed, and identify optimisation opportunities. Engage cross-functional teams in these reviews to maintain alignment.
This framework promotes a performance-driven culture and ensures that AI workflow automation is a continuous improvement journey—not a one-time fix.
Real-World Case Studies
Case studies bring measurement strategies to life. By examining how real companies implemented AI automation and tracked its success, we gain insights into what works—and why. These examples are based on publicly documented initiatives and demonstrate how defined metrics, clear goals, and the right tools can yield tangible improvements.
1. Suncorp Group: AI in Claims Processing
Challenge: Suncorp faced delays and inefficiencies in handling insurance claims due to manual processing.
Solution: The company integrated AI technology, including IBM’s Watson, into its claims management to streamline assessments and improve customer service.
Reported Outcomes:
- Accelerated processing time for motor insurance claims
- Improved accuracy in claims decision-making
- Enhanced customer experience through faster response times
Suncorp’s initiative reflects how AI-driven claims processing can reduce delays and improve efficiency when combined with clear process redesign.
2. Siemens: AI-Enabled Procurement Optimisation
Challenge: Siemens experienced inefficiencies in procurement validation and vendor data matching, which increased manual workload and slowed down cycle times.
Solution: Siemens partnered with UiPath to automate tax code validation in purchase orders and adopted Scoutbee’s AI-driven supplier scouting.
Reported Outcomes:
- Reduced manual intervention in procurement workflows
- Improved vendor data accuracy and sourcing efficiency
- Faster cycle times for purchasing decisions
These initiatives show how Siemens used AI for both back-end validation and supplier identification, enabling smarter and faster procurement processes.
3. Capgemini: Enhancing the Onboarding Experience
Challenge: Capgemini needed to improve consistency and compliance in onboarding across multiple global locations.
Solution: The company emphasised reinventing the onboarding experience using cloud-based HR automation, focused on user-friendly design and standardisation.
Reported Outcomes:
- Streamlined onboarding workflows across regions
- Improved task completion and documentation compliance
- Greater employee engagement during onboarding
Although specific metrics were not publicly disclosed, Capgemini’s digital HR transformation highlights the role of automation in improving cross-functional alignment and employee experiences.
How Cflow Helps You Measure Automation Success
Cflow is more than a workflow automation platform—it’s a measurement ecosystem. It blends AI-driven capabilities with built-in analytics to ensure every automated workflow is accountable, efficient, and continuously improving.
Why Cflow Is Ideal for Measuring Automation Performance
- Business Workflow Analytics: Provides visual dashboards to monitor KPIs like turnaround time, approval delays, SLA breaches, and throughput.
- Custom Dashboards: Personalize views by user role or department, so stakeholders only see what matters to them.
- AI Escalation Tracking: Monitor how often AI routes tasks to humans and why, helping fine-tune AI behaviour.
- Feedback Loop Management: Embed feedback mechanisms within workflows, allowing users to rate or comment on automation steps.
- Audit Trails: Full traceability of actions across the workflow to ensure transparency and compliance.
- Predictive Analysis: Uses historical trends to forecast potential bottlenecks or SLA violations.
Cflow eliminates the need for additional analytics platforms and makes automation success tracking accessible to any team. Its no-code interface ensures that business users—not just IT—can create, modify, and monitor workflows.
Decision-Maker’s Checklist
Before declaring your automation project a success, make sure you can confidently answer these questions:
- Are automation objectives clearly tied to measurable KPIs?
- Do you have reliable baseline data to compare results?
- Is your AI logic explainable and traceable in audit trails?
- Are real-time reports accessible to decision-makers at all levels?
- Have users reported better experience and efficiency after automation?
If any of these elements are missing, consider refining your measurement approach. Clarity and consistency are the foundation of sustainable automation success.
Conclusion
AI workflow automation has the potential to transform business operations—but only if its impact is consistently measured. Without data, automation becomes guesswork. By using platforms like Cflow, businesses can unify automation with insight—tracking performance in real time, correcting inefficiencies, and demonstrating clear ROI.
With built-in analytics, predictive reporting, and seamless user feedback loops, Cflow empowers businesses to manage automation like a strategic asset—not a technical experiment.
Sign up for Cflow today and start measuring the true success of your automation initiatives with confidence, clarity, and control.
FAQs
1. What are the key KPIs for AI automation success?
Turnaround time, cost savings, error reduction, escalation frequency, and user satisfaction are some of the most important metrics to track.
2. Can success be measured without expensive analytics tools?
Yes. Cflow offers built-in dashboards, custom reporting, and data exports—removing the need for costly third-party analytics platforms.
3. How often should AI workflow performance be reviewed?
It’s recommended to review AI automation performance quarterly, or immediately after workflow changes, to ensure consistency and adaptability.
What would you like to do next?
Automate your workflows with our Cflow experts.